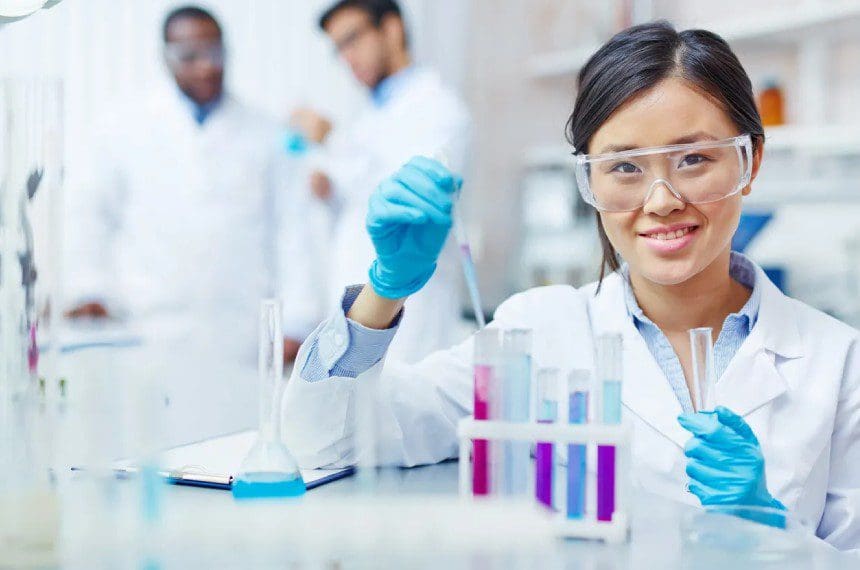
Laboratory Data Aggregation
By Pamela Noble MSHI MLS (ASCP) CM Laboratory data is an increasingly valuable tool in healthcare as organizations move towards the integration of data in health care information systems. A major challenge to this is in automating the aggregation of data from different sources. The core problem is that the code sets used in the underlying data files for laboratory test names differ from one electronic health information system to the next. When the data files are received by another provider, they cannot be interpreted. A solution to this problem is to map the local test names from these systems to a standard set of codes.
Pamela Noble MSHI MLS (ASCP) CM, a Health Systems Specialist and Senior Terminologist at J P Systems, answers questions on standardizing data for the lab domain.
Q: What are the problems with standardizing data for the lab domain?
There is little to no harmonization of the complete testing process. The data points that contribute to the testing process, such as terminology, sample quality, specimen type, units, reference ranges and test limits, report formats, and interpretation criteria, all need to be standardized. There are roadblocks to standardization, as the specifics of lab test results can vary widely from laboratory to laboratory. To establish a consistency which can lead to data harmonization, laboratory test data provided by different laboratories at different times on the same patient must be semantically equivalent. The COVID-19 pandemic has highlighted the need to share semantically equivalent laboratory data to diagnose and effectively monitor the spread to improve patient outcomes and identify outbreaks.
Q: What are the current issues in lab terminology?
There is an emphasis on interoperability in laboratory data and a need to codify more than just lab test orders and results. By using clinical terminologies and ontologies like LOINC, CPT, and SNOMED to standardize lab data, we can advance the foundations for the reliable use of machine learning and artificial intelligence to provide robust laboratory data analytics. Many laboratories do not have the resources to do this, but companies like J P Systems have the domain expertise to support such efforts.
Q: What is at the forefront of people’s minds, and what do they want to learn?
They want to learn how to effectively harness lab data and implement advanced data analytics to improve quality, decrease costs, test utilization, improve revenue capture, and decrease testing errors.