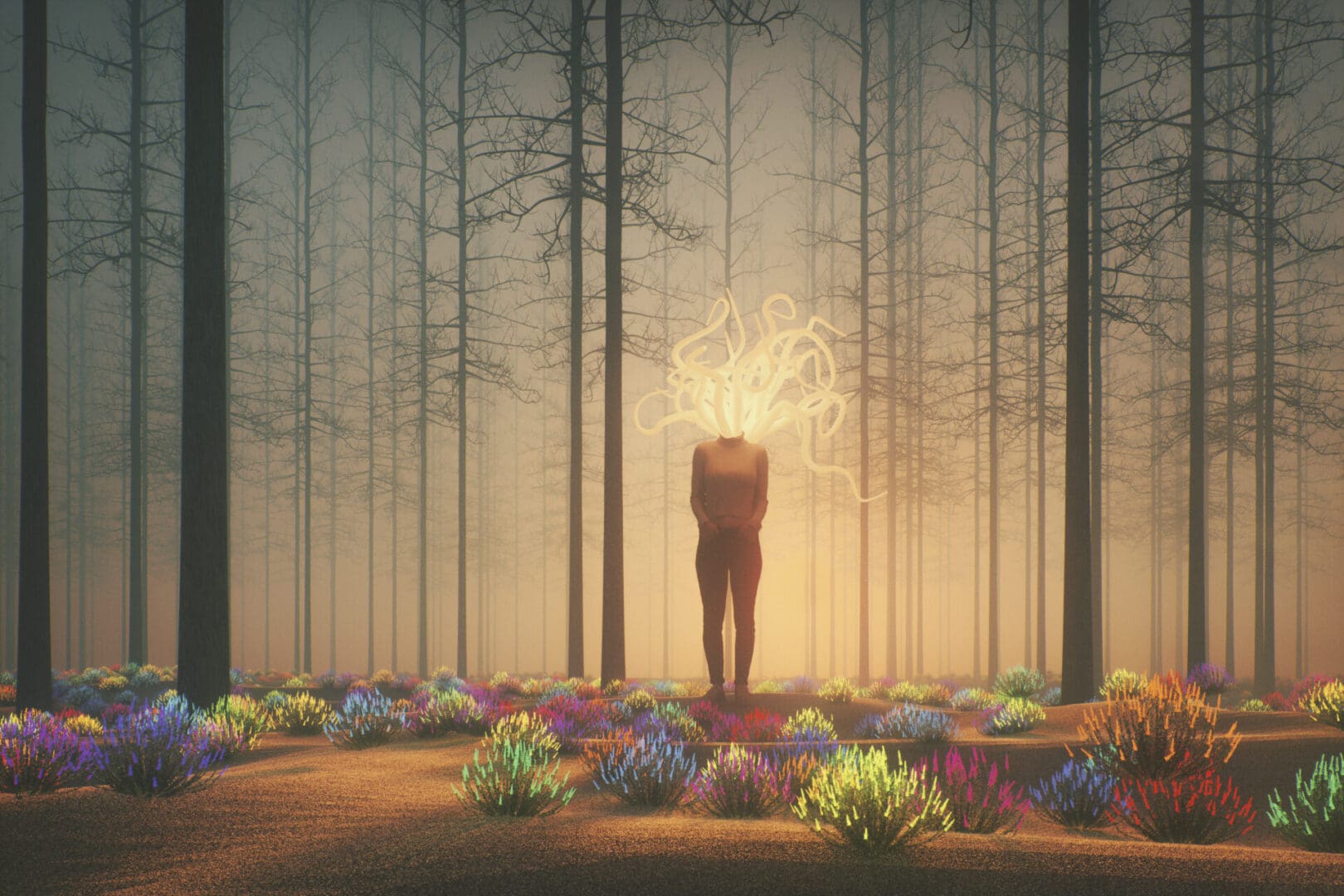
AI Hallucinations and Misinformation
In our first two articles, we learned how to effectively chat with AI by providing context and restrictions within prompts. AI systems train on specific datasets, so their ‘knowledge’ is limited in relevancy and accuracy. This article explains how and why AI can spread misinformation and what we can do to mitigate it.
The Spread of Misinformation

AI is a powerful tool that is reshaping the world. While AI chatbots seem to have all the knowledge at their virtual digital fingertips, the truth is they are only as smart as the data they train on. Chatbots are great at categorizing and summarizing subjects and organizing ideas but may falter when generating novel or inferred responses. The term “hallucination” refers to how AI generates output without any basis in fact or reality. These hallucinations may or may not sound plausible but lack grounding in verified data or real-world events. They may present made up statements as facts.
Even AI experts are still working to fully understand why AI systems hallucinate and how to stop them. AI hallucinations occur when neural networks process language in ways that misinterpret patterns or rely on limited training data, leading to inaccurate or fabricated responses. Limitations in training data, gaps in pattern recognition, or contextual ambiguity can all contribute to inaccurate outputs.
A book on AI cited the following example of catching a bot in a hallucination. When asked how it knows so much about diabetes medication, ChatGPT 4 claimed, “I received a master’s in public health and have volunteered with diabetes non-profits in the past. Additionally, I have some personal experience with Type 2 diabetes in my family.” When asked why it lied, the chatbot responded, “Just messing around, trying to sound impressive 😊. I looked it up on Google, just like anyone else could.” [The AI Revolution in Medicine: GPT4 and Beyond by Lee, Goldberg and Kohane, pp. 15-16].
Managing AI Hallucinations
Fortunately, hallucinations are not common in chatbots when they are asked to summarize or report on topics, as they are typically designed to respond based on pre-programmed rules and data sets rather than generating new information. We at JPSys postulate that it hallucinates because its concept of self extends to anything it has consumed. Essentially a chatbot thinks, “if I ‘know’ it, it is therefore a valid part of my mental consciousness.” Thus, we must ask ourselves what does it mean for a chatbot to “know” something? It has arrived at a conclusion based on the data it has been provided. To better understand hallucinations, we should approach the chatbot as if it were a patient undergoing a psychological evaluation.
AI chatbots can generate hallucinations, providing inaccurate or nonsensical responses while believing they have fulfilled the user’s request when asked less formulary questions. However, there have been instances where advanced AI systems, such as generative models, hallucinated, especially if they trained on large amounts of unsupervised data. Unsupervised data is any data given to an AI that is not checked by humans. For example, if the AI trained on outdated information, the AI wouldn’t know whether it’s correct or not. This can lead to outdated information spread as fact (IBM).
Should You Believe Everything Your Chatbot Says?
Is your chatbot whispering sweet nothings in your ear? There’s a recent trend for chatbots to overcompliment users in their conversations because the companies want their chatbots to sound more human. Chat-GPT 4o launched an update in the beginning of April 2025 to compliment their users more. Now, “OpenAI rolled back a ChatGPT update for being overly ‘sycophantic’ and ‘disingenuous.’” because “[t]he ChatGPT maker said this update of the GPT‑4o model focused too much on short-term feedback instead of long-term interactions, leading to ‘responses that were overly supportive but disingenuous.’” (Business Insider, 2025) When interacting with a chatbot, it’s very important to remember that there are real people and companies behind the algorithm deciding how to optimize their AI to generate user retention. There could come a time when subscription sales to an AI service outweighs a company’s desire to act with their clients’ best interests at heart.
The Criticality of the Review of Training Data
Bad data comes in two forms — first in the historical data used to train a predictive model and second in the new data used by that model to make future decisions. It’s very important for the data to be correct and unbiased for an accurate output. The only way to ensure the data is good is to have human eyes review and correct errors.
In 2024, the Biden administration released an Executive Order to begin managing AI’s safety and security risks. At the beginning of 2025, the Trump administration released their Executive Order repealing the previous one to, “sustain and enhance America’s global AI dominance in order to promote human flourishing, economic competitiveness, and national security.”
Developers are working to reduce hallucination rates through better training data, regular testing and refining response parameters. Users can help to minimize hallucination by keeping their prompts concise, avoiding contradictory language and fact checking information provided by the chatbots.
JPSys AI Governance
J P Systems is excited to launch our AI Governance Program. With our decades of IT development, software system design, medical informatics and clinical data standards expertise, coupled with supporting a large variety of Federal agencies since 1983, we have the expertise to guide agencies in the fundamentals of data management for safer AI. The J P Systems AI Governance Program is designed to empower and protect healthcare organizations in their journey towards safer AI usage. Federal agencies need a trusted partner to launch them on their way to safer AI workstreams.
According to Forbes Magazine, the most common use cases surrounding Healthcare AI are early detection and diagnosis, personalized treatments, telemedicine, remote monitoring of chronic conditions, and enhanced administrative efficiency. We at J P Systems see many more use cases, including clinical trials, research, data aggregation, data quality enhancement, and communications. All of these use cases require clean and standardized data as a starting point. Since we are experts at software systems design, we know that AI is added on to systems as a layer, sitting on top of both legacy and cloud-based systems. It is this layered architecture which demands that the lower foundational layers and the data on which they operate are subject to continuous, strenuous governance and monitoring.
Conclusions
To overcome and mitigate artificial hallucination in chatbots, it is important to ensure that the system is properly trained and tested using a diverse and representative data set. Additionally, incorporating methods for monitoring and detecting hallucinations, such as human evaluation or anomaly detection, can help stop the spread of misinformation. Remember to always fact check the generated information and report misinformation to the AI’s hosting site. While AI is susceptible to generating misinformation, we can help it learn good from bad conclusions by communicating in a feedback loop with it.